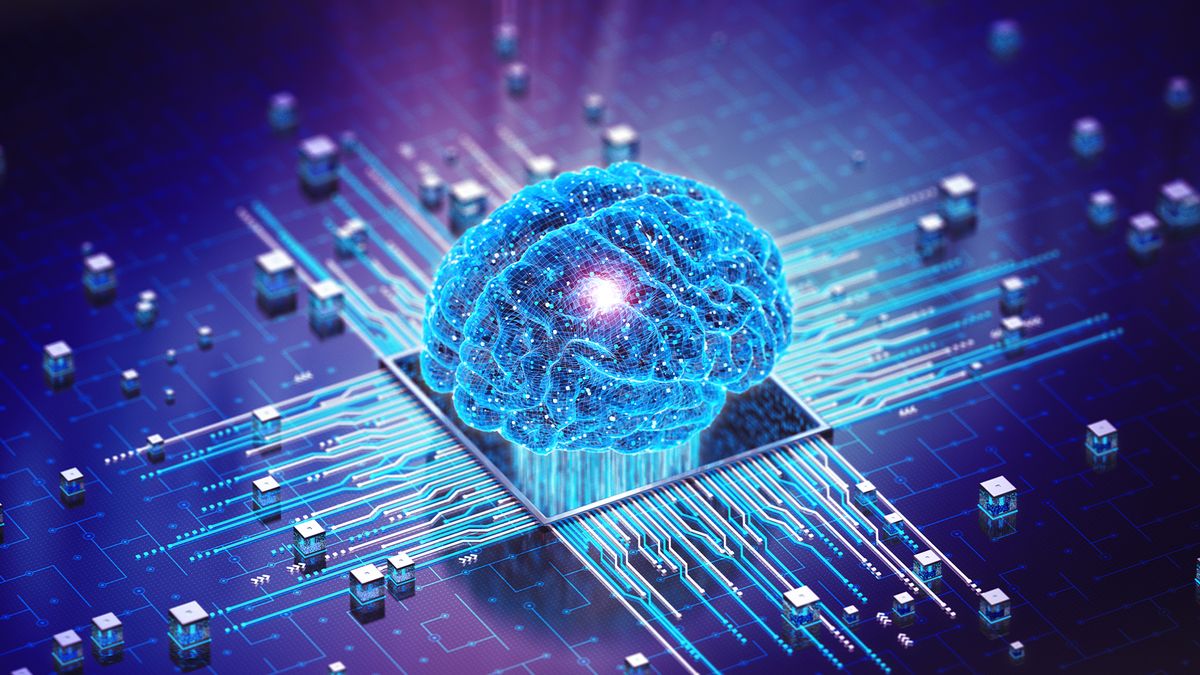
Why AI Models Fail in the Real World
Artificial Intelligence has dazzled us with its promise of transforming industries, automating tasks, and even mimicking human intelligence. From voice assistants to self-driving cars, AI models appear to be everywhere. Yet, despite impressive advancements, these models often stumble when faced with the messy, unpredictable reality outside the laboratory. Why do AI predictions sometimes fall flat in the wild? Let’s embark on a curious journey to uncover the quirks and quandaries behind AI’s real-world challenges.
When Predictions Go Wrong: The Curious Case of AI in Reality
Imagine teaching a child to recognize animals using pictures, and then surprise—when shown a different angle or a new species, the child struggles. Similarly, AI models often perform remarkably well on their training data but falter when faced with unfamiliar inputs. This phenomenon, known as overfitting, occurs because models become too tailored to specific datasets, lacking the flexibility to generalize beyond what they’ve seen. The real world, brimming with noise and variability, exposes these gaps, leading to unexpected errors.
Another culprit is the unpredictability of real-world environments. Data collected in controlled settings (like lab tests) rarely captures the chaos of everyday life—think of a self-driving car encountering a unexpected roadwork or a facial recognition system facing diverse lighting and angles. These variations, often called domain shifts, cause models to lose their predictive edge. As a result, AI systems that excel in test scenarios can stumble when the rules of the game change outside the lab’s safe boundaries.
Moreover, AI models sometimes lack common sense or contextual understanding, which humans instinctively apply. For example, an AI might misinterpret sarcasm or fail to grasp cultural nuances, leading to inaccurate predictions. While humans effortlessly navigate subtleties, AI models rely on patterns learned from data, which may not encompass the richness of human communication. These gaps highlight that AI, despite its brilliance, still misses the intuitive grasp of the world that we often take for granted.
From Lab to Life: Unraveling AI’s Hiccups in the Wild
Transitioning from a controlled environment to the unpredictability of real-world settings is a major hurdle for AI development. Labs often use curated datasets that are carefully labeled and balanced, but real life is messy and unpredictable. When AI models trained on such pristine data face the unpredictable chaos of real-world scenarios, their performance can degrade sharply. This ‘reality gap’ is akin to a perfect recipe that doesn’t taste quite right when served in everyday life.
One significant challenge is data bias. Training datasets may inadvertently reflect societal prejudices or lack diversity, causing AI to perform poorly for certain groups or situations. For instance, facial recognition systems trained predominantly on images of one ethnicity may struggle to accurately identify individuals from other backgrounds. Such biases not only undermine the model’s reliability but also raise ethical concerns about fairness and inclusivity. Addressing these biases requires careful, ongoing curation of training data and awareness of the models’ limitations.
Finally, the complexity of real-world environments makes it difficult to anticipate every possible scenario an AI might encounter. Unlike the predictable parameters of a laboratory, the world constantly throws curveballs—unexpected objects, new languages, or unforeseen behaviors. Building AI that can adapt on the fly, learn from new data, and handle ambiguity remains a formidable challenge. As researchers continue to innovate, the goal is to create models that are not just powerful but also resilient, versatile, and ready to thrive beyond the lab.
While AI models have made incredible strides, their journey into the wild remains a captivating adventure filled with surprises. From issues of overfitting to the messy unpredictability of real life, these challenges remind us that AI is still a work in progress—an evolving masterpiece that learns, adapts, and improves. By understanding why AI sometimes falters, we can better design systems that are robust, fair, and truly helpful in the diverse tapestry of the world. After all, the best AI isn’t just smart—it’s resilient, relatable, and ready for the real-world adventure ahead!